Applications
Archives
DIE
OCR
Revolutionizing Archival Document Processing with AI: Enhancing Degraded Historical Records
How can AI help us read and preserve history from severely degraded documents? This question drives our recent innovations at the Rényi AI group, where, in collaboration with the Historical Archives of the Hungarian State Security and the National Archives of Hungary, we’re pioneering methods to unlock access to aged historical records. By developing an advanced Document Image Enhancement (DIE) solution, we tackle challenges like faded text, ink bleed-through, and textural noise that even state-of-the-art OCR systems struggle with. Our approach significantly boosts text recognition accuracy, transforming archival work and empowering researchers in the field.
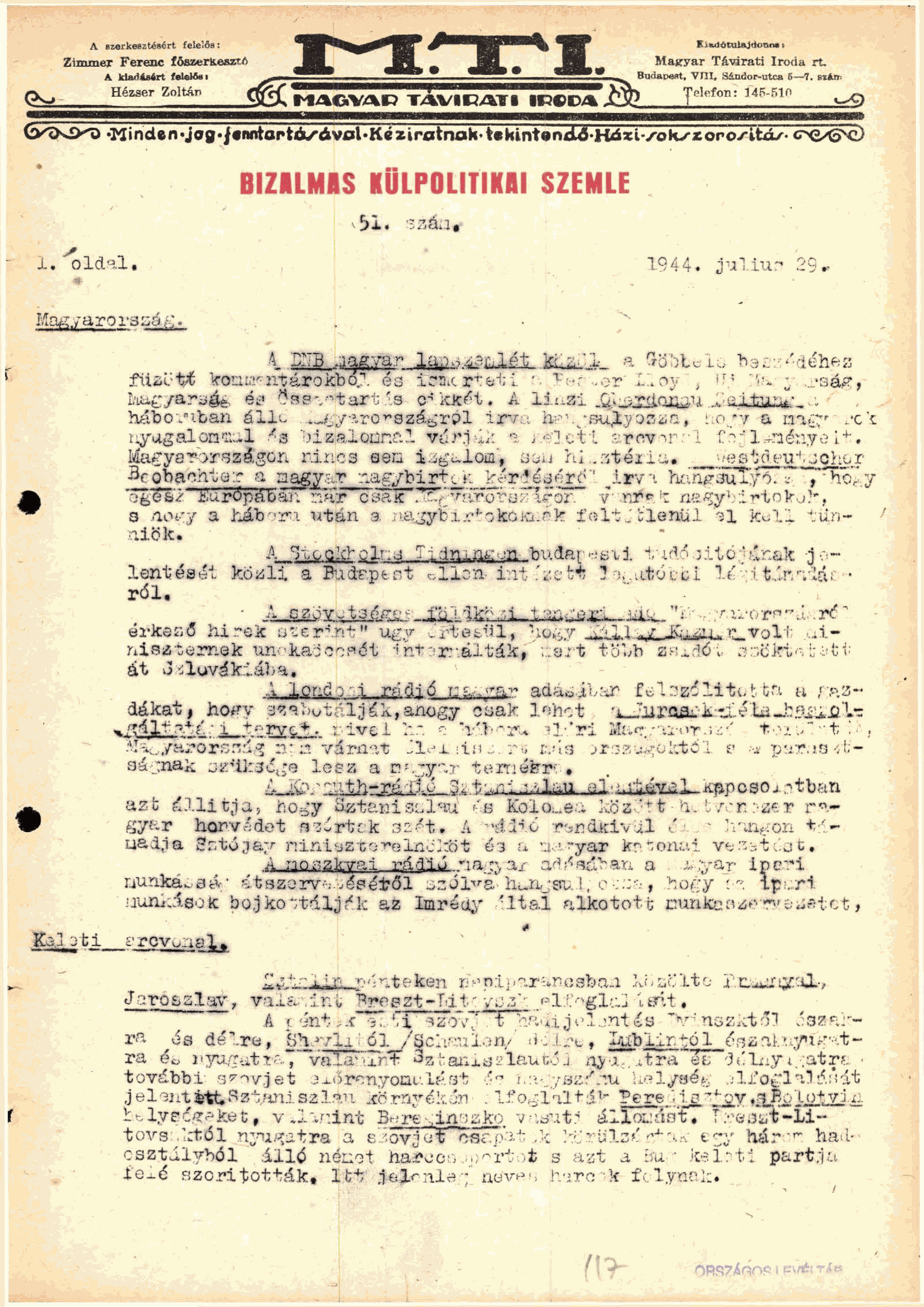
How a degraded historical document from the National Archives of Hungary is restored by our Document Image Enhancement model (DIE) model.